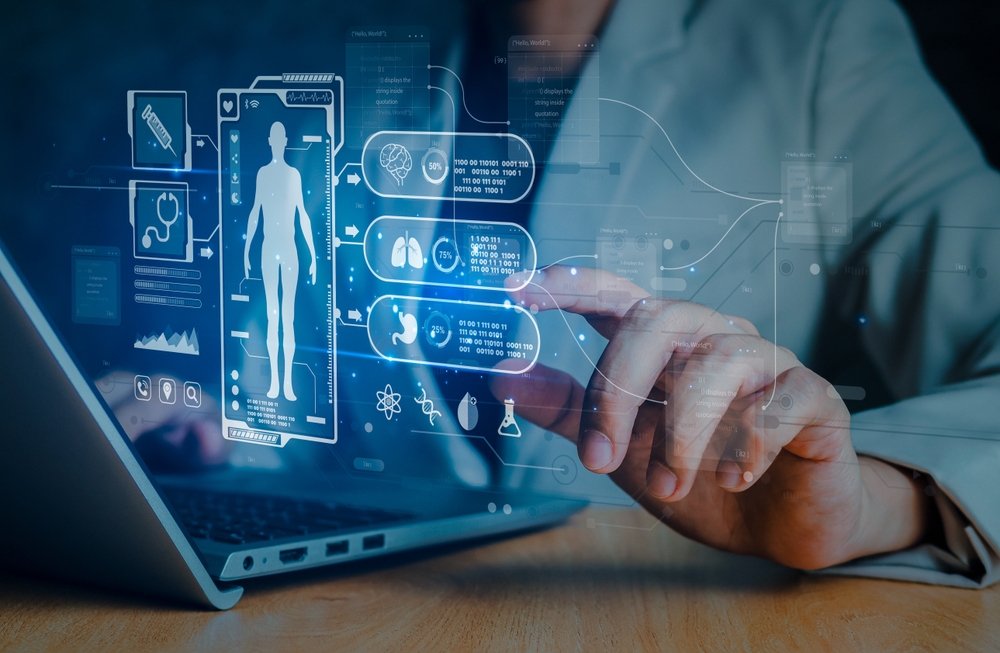
As artificial intelligence (AI) becomes more embedded in health care, the ability to accurately evaluate AI models is critical. In medical applications, where early diagnosis and anomaly detection are often key, selecting the right performance AI metrics can determine the clinical success or failure of AI tools. If a health care AI tool claims to predict disease risk or guide treatment options, it must be rigorously validated to ensure its outputs are true representations of the medical phenomena it assesses. In evaluating health care artificial intelligence, two critical factors, validity and reliability, must be considered to ensure trustworthy AI systems.
When using medical AI, errors are inevitable, but understanding their implications is vital. False positives occur when an AI system incorrectly identifies a disease or condition in a patient who does not have it, leading to unnecessary tests, treatments, and patient anxiety. False negatives, on the other hand, occur when the system fails to detect a disease or condition that is present, potentially delaying critical interventions. These types of errors, known as Type I and Type II errors, respectively, are particularly relevant in AI systems designed for diagnostic purposes. Validity is crucial because inaccurate predictions can lead to inappropriate treatments, missed diagnoses, or overtreatment, all of which compromise patient care. Reliability, the consistency of an AI system’s performance, is also substantially important. A reliable AI model will produce the same results when applied to similar cases, ensuring that physicians can trust its outputs across different patient populations and clinical scenarios. Without reliability, physicians may receive conflicting or inconsistent recommendations from AI health care tools, leading to confusion and uncertainty in clinical decision-making.
A physician must focus on three important AI metrics: 1) area under the precision-recall curve (AUPRC), 2) area under the receiver operating characteristic curve (AUROC), and 3) average precision (AP), and how they apply to health care AI models. In health care, many AI predictive tasks involve imbalanced datasets, where the positive class (e.g., patients with a specific disease) is much smaller than the negative class (e.g., healthy patients). This is often the case in areas like cancer detection, rare disease diagnosis, or anomaly detection in critical care settings. Traditional performance metrics may not fully capture how well an AI model performs in such situations, particularly when the rare positive cases are the most clinically significant.
In binary classification, where an AI model is tasked with predicting whether a patient has a certain condition or not, choosing the right metric is crucial. For instance, an AI model that predicts “healthy” for nearly every case might score well on accuracy but fail to detect the rare but critical positive cases. This makes AI metrics like AUPRC, AUROC, and AP particularly valuable in evaluating how well an AI system balances identifying true positives while minimizing false positives and negatives.
Area under the precision-recall curve (AUPRC) is a performance metric that is particularly well-suited for imbalanced classification tasks, such as health care anomaly detection or disease screening. AUPRC summarizes the trade-offs between precision (the percentage of true positive predictions out of all positive predictions) and recall (the percentage of actual positive cases correctly identified). It is especially useful in scenarios where finding positive examples, such as identifying cancerous lesions or predicting organ failure, is of utmost importance.
AUPRC is particularly relevant in AI health care because precision is critical, especially when treatments or interventions can have negative consequences. Recall is essential when missing a true positive, such as a missed cancer diagnosis, could be life-threatening. By focusing on these two AI metrics, AUPRC provides a clearer picture of how well an AI model performs when the goal is to maximize correct positive classifications while keeping false positives in check. For example, in the context of sepsis detection in the ICU, where early and accurate detection is crucial, a high AUPRC indicates that the AI model can identify true sepsis cases without overwhelming clinicians with false positives.
While AUPRC is valuable for evaluating AI systems in imbalanced datasets, another common AI metric is the area under the receiver operating characteristic curve (AUROC). AUROC is often used in binary classification tasks because it evaluates both false positives and false negatives by plotting the true positive rate against the false positive rate. However, AUROC can be misleading in imbalanced datasets where the majority class (e.g., healthy patients) dominates the predictions. In such cases, AUROC may still give a high score even if the AI model is performing poorly in detecting the minority positive cases.
For example, in a cancer screening program where the prevalence of cancer is very low, an AI model that predicts “no cancer” for most cases could still score well on AUROC despite missing a significant number of true cancer cases. In contrast, AUPRC would give a more accurate reflection of the model’s ability to find the rare positive cases. That said, AUROC is still valuable in situations where both false positives and false negatives carry significant costs. In applications like early cancer screening, where missing a diagnosis (false negative) can be just as costly as over-diagnosis (false positive), AUROC may be a better choice for evaluating AI model performance.
Another important AI metric is average precision (AP), which is commonly used as an approximation for AUPRC. While there are multiple methods to estimate the area under the precision-recall curve, AP provides a reliable summary of how well an AI model performs across different precision-recall thresholds. AP is particularly useful in health care applications where anomaly detection is key. For instance, in predicting hypotension during surgery, where early detection can prevent life-threatening complications, the AP score provides insight into the AI system’s effectiveness in catching such anomalies early and with high precision.
There are different ways to estimate the area under the precision-recall curve (AUPRC), with the trapezoidal rule and average precision (AP) being two of the most common. While both methods are useful, they can produce different results:
For AI health care applications like cardiac arrest prediction, where precise detection is vital, AP often gives a clearer picture of the AI model’s ability to balance precision and recall effectively. Physicians must be aware that in health care, making clinical decisions based on AI predictions requires a deep understanding of how well the AI model performs in rare but critical situations. AUPRC may be suited to evaluating AI models designed to detect rare conditions, such as cancer diagnosis, sepsis detection, and hypotension prediction, where a high AUPRC score ensures that the AI system is catching these rare events while minimizing false alarms that could distract clinicians.
In summary, the evaluation of AI models in health care requires careful consideration of which AI metrics provide the most meaningful insights. For tasks involving imbalanced datasets common in health care applications such as disease diagnosis, anomaly detection, and early screening, AUPRC offers a more targeted and reliable assessment than traditional AI metrics like AUROC. By focusing on precision and recall, AUPRC gives a more accurate reflection of an AI system’s ability to find rare but important positive cases, making it an essential tool for evaluating AI in medical practice. Average precision (AP) also serves as a valuable approximation of AUPRC and can provide even more precise insights into how well an AI system balances precision and recall across varying thresholds. Together, these AI metrics empower clinicians and researchers to assess the performance of AI models in real-world health care settings, ensuring that AI tools contribute effectively to improving patient outcomes.
Neil Anand is an anesthesiologist.
Health IT
Get free updates delivered free to your inbox.
Search thousands of physician, PA, NP, and CRNA jobs now.
Learn more
Founded in 2004 by Kevin Pho, MD, KevinMD.com is the web’s leading platform where physicians, advanced practitioners, nurses, medical students, and patients share their insight and tell their stories.
Comments are moderated before they are published. Please read the comment policy.