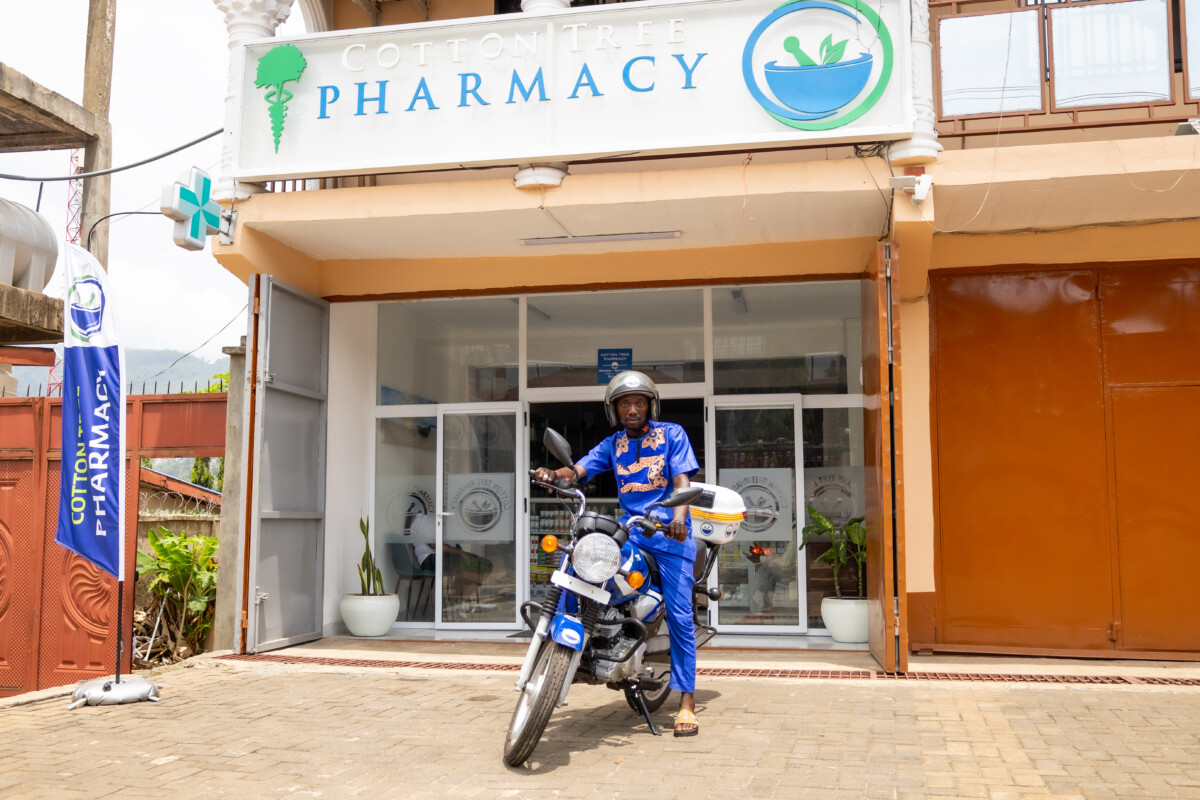
© Copyright Swit Salone. All Rights Reserved.
Cotton Tree Pharmacy has moved from the town center to a new location at 175 Regent Road in Malama. Customers can expect the same excellent service plus free delivery, for orders over Nle 230. However, there’s an ongoing promotional service so from now till the 1st of August 2024 there is a free delivery service for all orders. Our delivery service operates Mondays to Saturdays from 9:00 am to 7:00 pm, ensuring that you receive your medications and healthcare products in a timely manner.
Staff of the Cotton Tree Pharmacy
Reflecting on our journey, it’s incredible to see how far we’ve come. Six years ago, Dr. Fouad launched the first Cotton Tree Pharmacy on Pa Demba Road, with a vision to expand access to authentic medication. Today, we stand proud with three pharmacies, a testament to our success and the trust we’ve earned through our public-private partnership with the Ministry of Health.
Bariatu Barrie | Pharmacy Technician
At Cotton Tree Pharmacy, we’ve always been more than just a place to pick up prescriptions. We’re a community hub, a trusted source where patients can find genuine brand-name and generic products from reputable manufacturers worldwide.
“Our mission has always been and will continue to be, to meet the healthcare needs of Sierra Leoneans, both at home and abroad, because your health is our priority,” said Dr. Fouad Sheriff
Dr. Sheriff owns Cotton Tree; he established the pharmacy six years ago after discovering that 20-30% of the patients he treated during his first medical mission to Sierra Leone were using counterfeit medications for chronic ailments.
“I knew I wanted to make a difference by opening a pharmacy selling authentic drugs.
Cotton Tree Pharmacy represents our collective aspiration for a healthier and prosperous Sierra Leone,” says Dr. Sheriff.
His vision was a pharmacy that would serve the needs of his community in the most comprehensive way possible. The pharmacists at Cotton Tree Pharmacy Aminata Frederica Mansaray, pharmacist with five years of experience and Bariatu Barrie, who has worked as a Pharmacy Technician for less than a year, are there to help you whether you need medication, blood pressure management, or advice.
The move to 175 Regent Road Malama marks a significant milestone for Cotton Tree Pharmacy. The new location not only boasts a larger space, allowing for an expanded inventory. Patients can now expect the same high-quality products and personalized care, along with these exciting additions, in a more accessible and convenient setting.
Cotton Tree Pharmacy’s Pharmacist & Pharmacy Technician:
From Left to Right; Aminata Frederica Mansaray & Bariatu Barrie
One of Cotton Tree Pharmacy’s unique aspects is its ability to serve Sierra Leoneans at home and abroad.
“No matter where you are, if your family is here and unwell, you want to do anything to help them,” said Dr. Sheriff.
“That’s where we come in. We can deliver medicines to anyone anywhere in Freetown and beyond. Payments can be made online, and we can dispense the very same day.”
They have leveraged technology to better provide efficient delivery services online, such as ordering and paying for medication and offering free delivery in Freetown. This service is a testament to the pharmacy’s commitment to bridging the gap between loved ones and ensuring that healthcare is accessible, regardless of distance.
Cotton Tree Pharmacy New Location at 175 Regent Road, Malama
The entire team at Cotton Tree Pharmacy invites you to visit their new location at 175 Regent Road in Malama. We are open from Mondays to Saturdays at 9:00 am till 7:00 pm. Whether you need to fill a prescription, consult with a pharmacist, or purchase healthcare products, you can trust Cotton Tree Pharmacy to meet your needs with the same dedication and care that has defined their service since 2018.
Join us in celebrating this new chapter and continue to rely on Cotton Tree Pharmacy as your one-stop healthcare destination. We remain committed to providing the same level of service and satisfaction that you have come to expect from us. For more information, visit our website at cottontreemedicalgroup.com or call +232 30 611664/+232 73 820782 or walk in to our new location on 175 Regent Road. We look forward to serving you at our new home.
Comments are closed.
Subscribe to our mailing list to receives daily updates direct to your inbox!